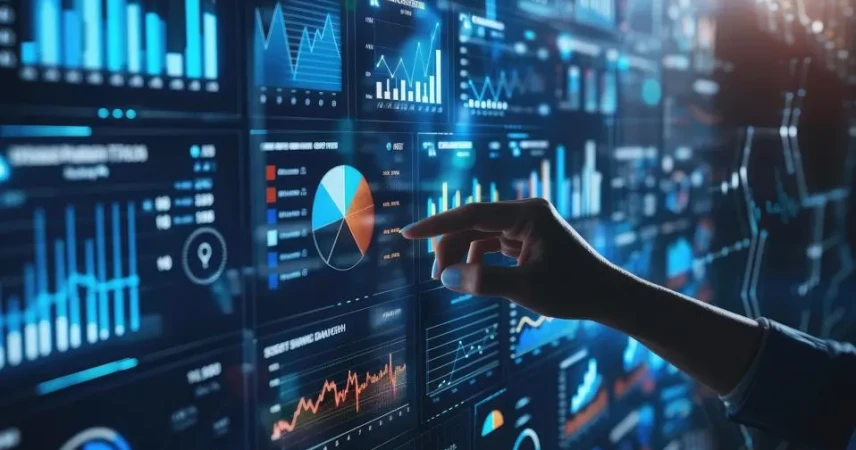
17 Generative AI Data Analytics Tools Everyone Should Know About
Thanks to generative AI, we’re getting close to the promise of truly “democratizing” data. This means anyone can make decisions that are data-driven, not just highly skilled data scientists.
Here ‘s the issue: The amount of data in the world is growing exponentially. And hidden within all of that information are insights that can potentially help us do anything from curing cancer and discovering new sources of clean energy, to improving our health and fitness and growing our businesses.
So the problem isn’t that we don’t have enough information do these things. It’s that we have too much, and don’t know the best place to start!
Traditionally, extracting insights and value from data required two things – very clever, highly trained people and lots of time.
Here, AI has proven very useful. For the past decade, we’ve used deep learning algorithms to crunch numbers far quicker than any human, spotting patterns and pulling out insights. It also allows us to find clues hidden in unstructured data, such as text, video and sound.
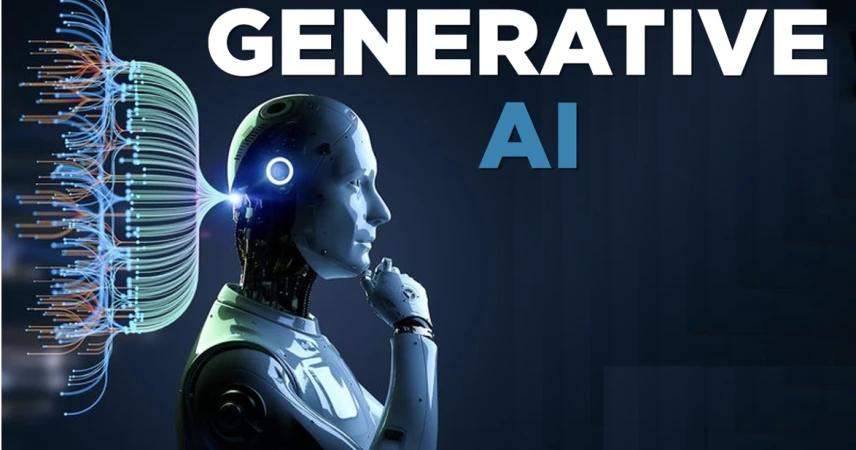
Built on its Alteryx AiDIN engine, the platform differentiates itself from competitors with a user-friendly approach to predictive analytics and insight generation. A key feature is its Workflow Summary Tool, which interprets complex workflows into simple-to-understand natural language explanations and summaries. You can also specify how you want your reports to be output, for example, in email or PowerPoint formats, to target your desired audience. This is an industry-standard analytics package that’s been enhanced with the power of generative AI, harnessing Microsoft’s Co-Pilot technology and models developed with OpenAI, including customized versions of its powerful GPT-4 models.
The platform integrates numerous technologies including Microsoft’s Fabric AI-powered analytics framework, as well as Azure Synapse which allows it to seamlessly integrate with data warehouse and other Big Data technologies like Apache Spark. This creates an end-to-end analytics solution suitable for the largest enterprise workloads, enabling anything from simple analytics to building and deploying your own machine learning models on Azure. It’s a good choice for enterprise users needing flexible cloud integrations and dealing with extensive data workloads.